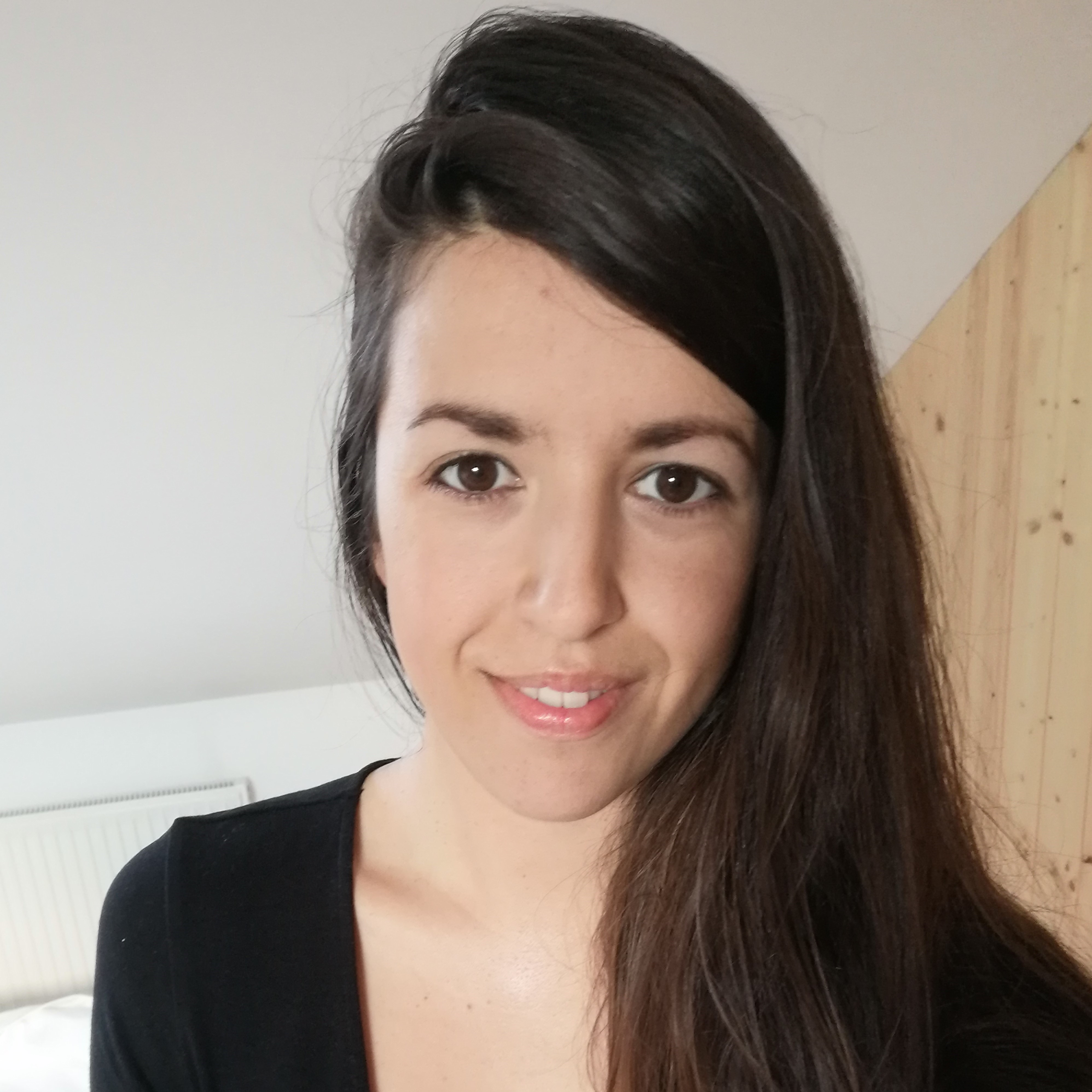
Mica Komarnyckyj
Mica is an addiction neuroscience researcher with a background in engineering and R&D technology funding and commercialisation. Her current research project at the University of Huddersfield aims to develop a drug testing platform based on machine learning single trial EEG analysis and ERP techniques, with the initial application of treatments for alcohol dependency. Her prior research utilised the same technique to differentiate young adults at-risk for alcohol dependency from those with normal drinking behaviours. She also has experience working with fMRI data, conducting an exploration of craving in opioid dependency using psychophysiological interaction (PPI) connectivity analysis.
PhD Symposium: At-risk alcohol users have disrupted valence discrimination during reward anticipation
Alcohol dependency (AD) is characterised by disrupted reward processing, underpinned by dysfunctional cortico-striatal reward pathways. The distinct alterations within this reward circuitry regulate the development and persistence of this disease. While an abundance of research has identified the biomarkers of AD, little is known about the neural markers of disrupted reward processing in populations who are at-risk of AD, which could facilitate more targeted prevention and intervention strategies. Here we used an electroencephalography (EEG) version of the monetary incentive delay task to clarify whether young adults with high alcohol use have disrupted reward anticipation. The Alcohol Use Disorders Identification Test (AUDIT) was used to quantify at-risk alcohol use, with participants split into high alcohol (HA) (n = 22, mean AUDIT score: 13.82) and low alcohol (LA) (n = 22, mean AUDIT score: 5.77) groups. We focused on two dimensions of reward anticipation: valence (i.e. triggering approach or avoidance behaviour, gain vs loss) and motivational salience (i.e. stimulating the need to take action, incentive vs neutral). In addition to conventional Event Related Potential (ERP) analyses – and to reliably disentangle the valence and salience dimensions – we also applied machine learning multivariate single-trial classification to the EEG data. In the early stages of reward anticipation after cue presentation (250 – 550ms), HA group evidenced a blunted neural response to the valence dimension of reward anticipation, aligning with prior fMRI findings in AD. Conversely, the LA showed both significant ERP-P3 differences as well as high machine learning discrimination between Gain and Loss anticipation. At the later stage of reward anticipation, during preparation for motor response to the target, we found a differential signal across the groups along the salience dimension, with a higher discrimination for the HA group, reflecting altered motivational mechanisms in the at-risk population.
Click here for presentation slides.