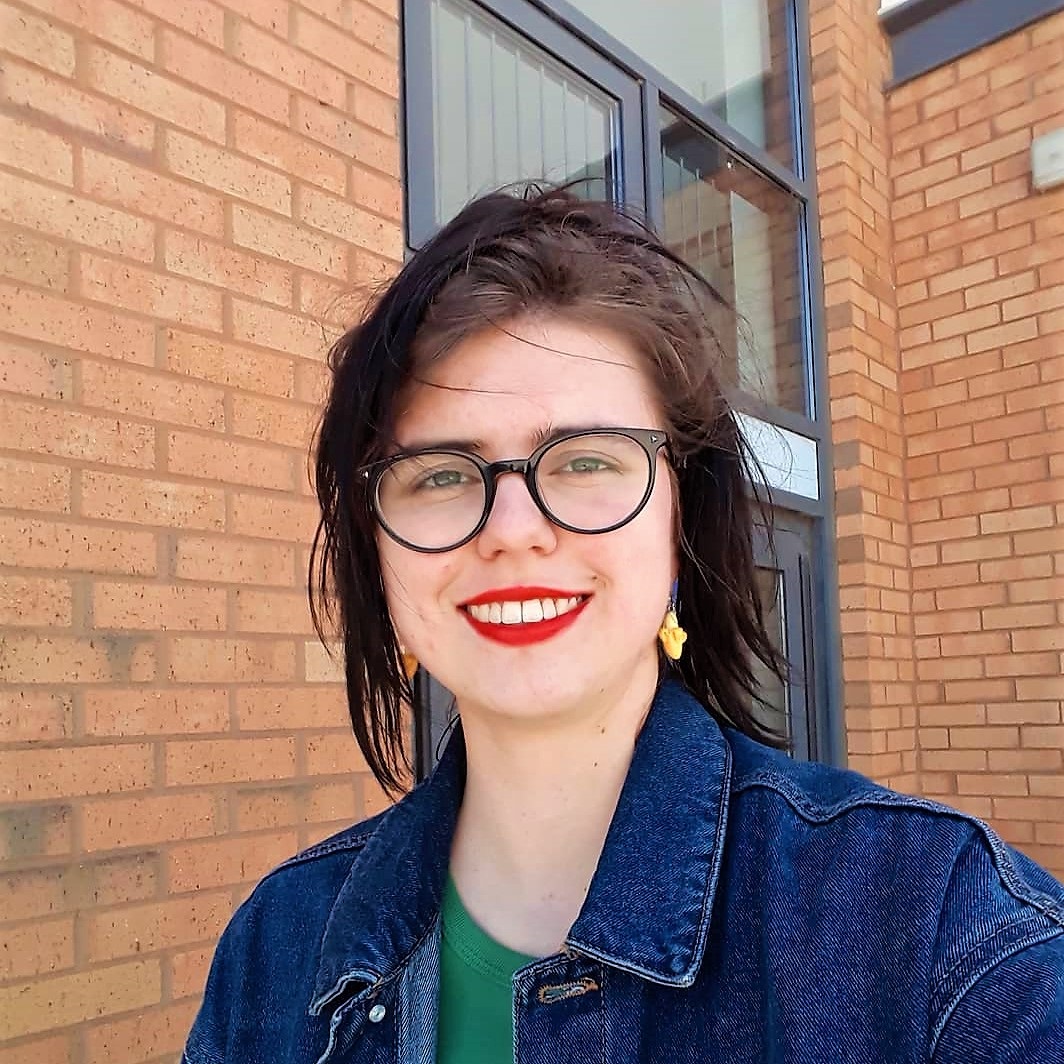
Kristina Hnízdilová
Kristina Hnízdilová is a PhD Student at the University of Dundee after completing her undergraduate and postgraduate studies in Psychology at the University of Stirling.
Prior to beginning her PhD studies in September 2021, she worked as a research assistant at the Salvation Army Centre for Addiction Services and Research at the University of Stirling, being involved in studies related to digital inclusion, overdose prevention interventions and Housing First.
Her research interests include harm reduction, homelessness, qualitative research methods and overdose prevention.
After completing her PhD, she intends to continue to work in academia.
The RESCU Project: Piloting a digital healthcare respiratory monitoring intervention to reduce drug-related deaths
Delegate Poster Prize-winner (student-led), 2022: Best inclusion
Aims
Opioid-induced respiratory depression causes most drug-related deaths. This study investigates whether a chest-worn accelerometer sensor can dependably capture respiratory patterns of people who use drugs to determine trigger points for an emergency response. The study assesses device acceptability to people who use drugs and stakeholder groups to create an intervention pathway.
Design
RESCU is a mixed-methods observational cohort study with a planned duration from January 2022 to January 2023.Setting Participants are recruited on a rolling basis from a needle exchange in the city centre of Dundee.
Participants
Participants (planned n=100) are individuals accessing services in NHS Tayside.
Intervention
Participants receive a sensor and a gateway device to passively monitor their breathing when in range, over a four week period. Participants record their drug use, returning to the exchange weekly for data download. Semi-structured interviews (planned n=20) and focus groups are conducted with participants who have completed the study protocol and stakeholder groups from the third sector support groups and first responders. Measurements During February – April 2022, 36 participants had been enrolled in the study. Data were reviewed after running prototype apnoea and movement artefact detection algorithms.
Findings and Conclusions
4,483 sleep apnoea episodes of >10s duration were detected at the highest level of probability in 1,748 hours of respiratory data. Current data suggests the device successfully captures respiratory anomalies. A future aim is to identify emergency response trigger points. Thematic analysis results and further data will be available by the time of the conference.
Poster link: The RESCU Project: Piloting a digital healthcare respiratory monitoring intervention to reduce drug-related deaths